For a wide range of companies, delivering quality rich media experiences is of paramount importance. However, measuring the quality of video that viewers actually experience has been difficult, if not impossible, in many circumstances where it is not possible to explicitly ask viewers to rate the quality of the video, or when a reference video is not available.
HeadSpin’s patent-pending reference-free video MOS or Mean Opinion Score provides a flexible, accurate, and scalable alternative to traditional survey-based and full-reference algorithmic approaches, using artificial intelligence (AI) to address the challenges of reliably assessing perceptual video quality.
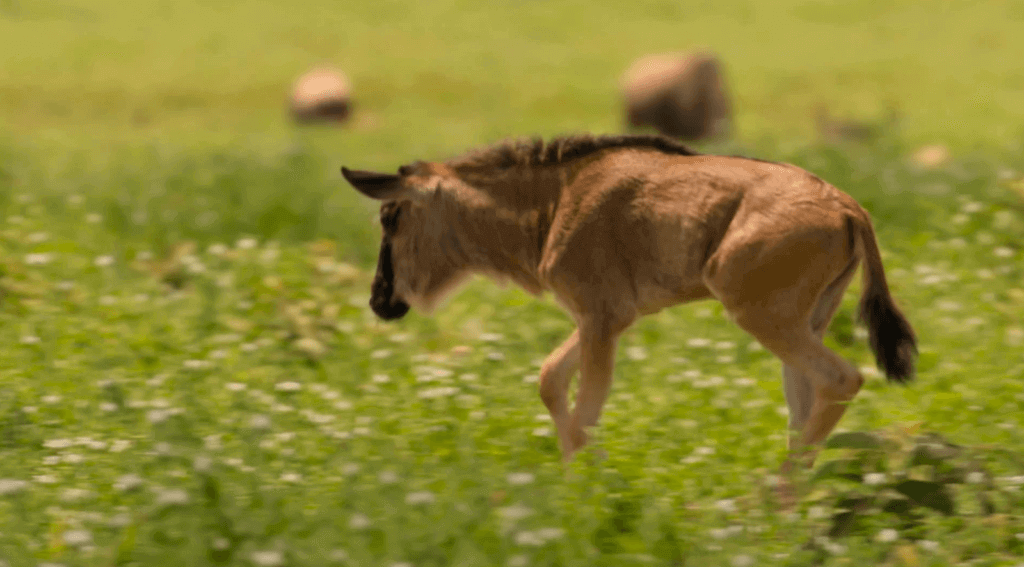
In high quality wildlife videos, the blurriness of the background is a deliberate result of focusing on the animal. This is an example of something an AI deep learning model could be trained to recognize but would be missed entirely by a parametric model based on video quality metrics.
Importance of Video Quality
Poor experiences matter. They lead to dissatisfied users, and dissatisfied users don’t stay dissatisfied for long—they go elsewhere. An Akamai report found that a single buffering event causes user happiness to drop by 14%. Conversely, higher bit rates can boost viewer engagement by more than 10%.
Today, rich media content, including live video streams on social platforms, video conference and chat services, mobile gaming, and television broadcasts, represents the lion’s share of network traffic and a major part of the user experience. Poor digital experiences could be caused by an issue with the video source, network conditions that cause packet loss and streaming delays, and even device-specific issues that create rendering problems. When experiences are suboptimal, any number of elements across a range of domains could be the culprit and it’s vital for organizations to be able to understand—and optimize—the quality of video content delivery on real devices in various locations, in real time.
Traditional Video Quality Monitoring Approaches
Video quality monitoring has traditionally been assessed using a metric known as the mean opinion score (MOS)—a subjective measure of perceptual video quality monitoring as rated by a jury of users. MOS scores are usually gathered in a quality evaluation test, such as when WhatsApp asks you to rate the quality of your video call, but they can also be algorithmically predicted in the absence of real user feedback.
For scenarios in which a reference video is available, there are existing standards that can be used to establish a video quality monitoring MOS:
- International Telecommunication Union Radiocommunication Sector (ITU-R). The ITU-R has well-defined standards for developing experiments to estimate video quality MOS. Using the standard, video quality MOS can be computed based on parameters derived from both the reference video and the test video. An estimate of the video MOS is calculated from a parametric model constructed using these video metadata parameters regressed against subjective quality scores from user studies.
- Video Multimethod Assessment Fusion (VMAF). VMAF is an open source algorithm developed by Netflix that uses a strict frame-by-frame comparison of reference and observed videos in order to make a prediction of the video quality MOS. This approach, while offering many benefits, is only meaningful across variants of a single reference video, e.g., multiple compressed versions of a video.
With both of these standards, teams are confronted with significant limitations.
- There are many scenarios in which it simply isn’t possible to establish a reference video. This is true in gaming scenarios, live video streaming, video calls, and a number of other cases in which content is dynamically generated.
- Reference videos are often too costly or resource-intensive to create and to maintain even if they may exist. This is often true in broadcast television and many video streaming scenarios.
- MOS scores from full-reference approaches cannot be meaningfully compared across different source files. They are typically only useful for a limited number of use cases, such as video compression optimization, when there is a need to compare across variants of a single reference video.
- Real world use cases often break fundamental assumptions in full-reference video MOS techniques. For example, even if the source video is the same, the screen recording of the video playback on the device is going to be slightly different for each playback. This was part of the motivation for our reference-free MOS.
- Many full-reference MOS scores are not very well grounded in the perceived quality. For instance, if the reference video itself is low quality, VMAF will produce a high score where a real user would not.
- Video technology and the consumer-facing content surrounding that technology are constantly evolving. The existing standards and solutions described above have no strategy for continuous improvement and evolution as technology standards continue to change.
These limitations pose significant challenges as teams continue to focus on delivering high quality rich media content.
Introducing the HeadSpin Reference-Free Video MOS
To address the challenges and limitations of traditional approaches to MOS, HeadSpin’s data science team developed a fully AI-based reference-free video MOS model leveraging experience from the 5+ years we’ve been in business. Our innovative patent-pending algorithm offers breakthroughs in the measurement of video quality by providing a reference-free estimate of the true subjective quality score of video content as perceived by end-users based on predictive machine learning models.
The reference-free approach enables test/QA and development teams to quickly and cost-effectively test, monitor, and analyze, at scale, the quality of video being delivered.
Key Features
Simple, Scalable Implementation for Better Video Quality Monitoring
HeadSpin’s MOS may be applied to any video content regardless of origin. It works seamlessly across devices, on videos captured directly on the platform, and even on third party videos imported to the platform through our API. A MOS score is estimated for every frame in the supplied video based on spatial and temporal features extracted from the video.
Video Quality Monitoring With Reference Free Analysis
Our reference-free approach enables our users to gauge video quality without any reference source video or comparison processes. In addition, the HeadSpin platform also offers a comprehensive suite of reference-free video quality metrics that track multiple video features such as blockiness, blurriness, and contrast. These additional metrics are presented in a time-aligned view alongside the video quality MOS time series and can be used to diagnose or explain regions that exhibit poor video quality scores. When paired with our expert system AI analysis, such as the Poor Video Quality Issue Card, the solution will surface insights into perceptual video quality issues, and into correlations between these issues and other app-related metrics.
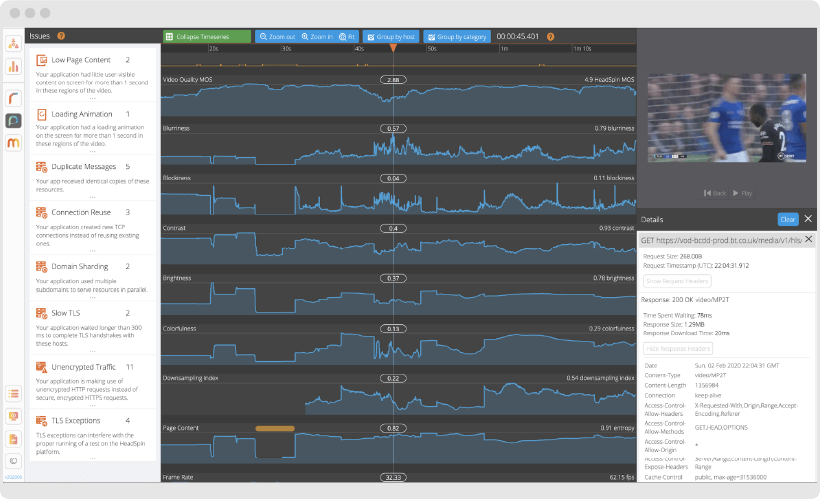
HeadSpin surfaces insights into perceptual video quality issues in a time-aligned view—highlighting correlations between frame-by-frame MOS scores and other video- and app-related metrics.
Improved Video Quality Monitoring With A Flexible, Accurate ML Model
Our reference-free video MOS is non-parametric and employs convolutional neural network technology to expose perceptual video quality features. It is the only MOS on the market that does not explicitly rely on other metrics for results. Unlike a parametric approach derived from video quality metrics (such as blurriness, blockiness, jerkiness, etc.), which is prone to false positives, for instance, from square or rectangular UI elements, splash screens, logos, stylistic pixelation, semi-transparent elements, and gaming special effects, our AI-based MOS has been trained to recognize these scenarios. In addition, the AI will accurately identify a video stream that is likely to be perceived as low-quality even if it is captured at high resolution. This unique approach gives HeadSpin the freedom and flexibility to capture and identify video quality issues that are not currently surfaced by existing standards like ITU-R and VMAF.
Unprecedented Wealth of Data for Accurate Video Quality Monitoring
HeadSpin has taken an innovative approach to leveraging our expertise and resources to create a model grounded in real world video streaming use cases so that the quality of the video can be estimated without a reference comparison. We curated the largest video quality data set of its kind consisting of thousands of unique videos captured under real-world conditions via the HeadSpin global device infrastructure. The AI model was trained on a subset of the videos captured and we have > 60,000 labels on > 700 videos from user studies. In terms of diversity of unique videos, diverse content from a diverse set of content providers, and sourcing labels from high quality labelers, our reference-free video MOS model is based on the most comprehensive dataset in the space.
Constant Improvement Toward Video Quality Monitoring With Continuous Optimization
Due to the nature of our deep learning architecture, our models continue to evolve. Incorporating data from the latest video streaming applications enables the machine learning model to accurately predict MOS on any video it analyzes. Continuously incorporating customer feedback into the model development process (via our Video Annotation App) enables us to improve the accuracy of the AI model over time.
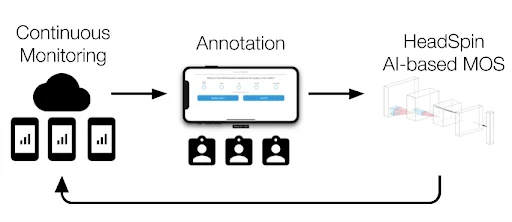
Better Together
HeadSpin additionally supports a full-reference VMAF MOS on the platform. While VMAF is more suitable for indicating degradation relative to the source, our reference-free MOS will indicate absolute video quality as perceived by the end user. Many of our users use both in tandem as complementary measures.
Video MOS Use Cases
Below are a few examples of the many ways our customers are employing HeadSpin’s video quality MOS.
Measuring Live Video Streaming
Live video streaming content is growing in popularity every day. More and more platforms are offering users the ability to both serve and consume live video content. Tracking the perceived quality of these live streaming videos is important to understanding how users perceive the quality of the platform itself. With its reference-free approach, the HeadSpin video MOS helps our users understand the video quality of live streaming content.
Reference-based Video Streaming Measurement
In cases where reference videos are available, HeadSpin’s deterministic AI algorithms can be used to compare a subsequent or parallel test against the reference to provide a comparison of the video quality. Users frequently set up systematic tests across devices, locations, and carriers, on repeated intervals, to get a better understanding of how these variables affect the perception of the video content being served from their platform.
Statistical Analysis
Our users frequently leverage our reference-free video MOS algorithm to aggregate the MOS over many experiments. This aggregated intelligence can be used to develop statistical methods for testing hypotheses. For example, they can use our AI platform to test a hypothesis that streaming video on a mobile app has greater variance in quality during peak internet usage than during non-peak times. HeadSpin can be used to instrument this experiment and collect the MOS data required to test this hypothesis.

To calibrate the accuracy of the human-annotated values used in our ML model, a rigorous exercise was conducted with a panel of data experts not involved in the annotation study, to come to a consensus on “ground-truth” MOS scores.
Conclusion
It is not uncommon for video content to receive millions of views every day. How these videos are perceived by the end user may rely on a multitude of factors, including content delivery network (CDN) configurations, video encoding and playback optimizations, network conditions, and more. In order to deliver better video hosting and serving services, it is vital to understand how different platform settings may impact the quality of video viewers see, and to understand how that experience may vary across devices and locations.
For many organizations today, video quality is too important to leave to chance or guesswork. With HeadSpin’s reference-free video MOS, app teams can gain the accuracy and scalability they need to track and optimize the quality of the viewers’ experience.
The HeadSpin Digital Experience AI Platform provides performance and quality-of-experience analytics for mobile, web, audio, and video. With HeadSpin, engineering, QA, operations, and product teams can assure optimal digital experiences throughout the development lifecycle.
Start measuring your video quality with reference-free ease today! Speak to a salesperson to get started.
FAQs
1. What can AI accomplish with video apps?
AI-based can recommend content or interactive stories. Data mining on end-user behavior enables game designers to investigate how players use the game, what areas they play the most, and what leads them to stop playing, allowing the developers to modify gameplay or enhance monetization.
2. What factors are considered while measuring video quality?
Factors that are considered to analyze video quality are:
- Bit Rate
- Buffer fill
- Lag length
- Play length
- Lag ratio
3. What elements are measured in video load testing?
With the help of an AI-backed video testing platform, it is possible to perform load testing for video apps and monitor:
- important video streaming metrics, including adaptive bitrate streaming
- real-time viewership and preparing for peak viewership scenarios
- viewing behavior of users and accordingly developing video streaming infrastructure
4. What are the HeadSpin AV platform features that help in testing video apps?
HeadSpin’s AI-powered AV solution has robust features such as:
- Cross-device and browser compatibility- for running tests on your OTT and other media applications like gaming, video conferences, and live streaming platforms across a wide range of devices.
- QoE & Streaming Performance KPIs- a comprehensive suite of reference-free quality metrics to analyze and check blockiness, blurriness, brightness, colorfulness, exposure, flickering, freezing, video frame rate drops, and buffering time
- Perceptual video quality analysis- based on predictive machine learning models to deliver accurate subjective analysis with a time aligned-view of video quality as perceived by end-users.